Consumption-based pricing has become a popular model for SaaS and PaaS businesses, allowing customers to pay only for what they use. Pioneers like Slack and AWS have successfully adopted this approach, offering flexibility and reducing waste. However, not all consumption-based models are created equal. The Monthly Active Users (MAU) model, while appealing in its simplicity, often leads to inefficiencies and unexpected costs. This article explores the strengths and weaknesses of various consumption-based pricing strategies, and shares the journey we have taken at Ably to identify a model that truly prioritizes customer value.
Consumption-based pricing we know and love that works
Consumption-based pricing is ubiquitous for SaaS and PaaS businesses today, and it’s not surprising. Conceptually it’s a big win for customers. Consume what you need and pay in arrears for what you have consumed, as granularly and accurately as possible.
Slack innovated in this area and took the traditional seat-based licensing models further by only charging customers for active seats in the month. This was a big departure from the traditional seat model, where their customers were not really paying for what they used, but for what they thought they would use. Slack’s small change was a huge win for customers as they can more freely issue licenses to their staff members without the fear of paying for software licenses that aren’t used.
AWS too has paved the way for effective consumption-based pricing and fundamentally changed the way developers rent server capacity. Traditionally, developers would choose an instance type that bundled a fixed amount of CPU, disk, memory and bandwidth, and be charged monthly. AWS changed that by letting customers dynamically configure what type of server capacity they wanted, and be billed by the second for the resources they consumed, with the lowest level of granularity for each resource, such as GB for disk storage.
Both Slack and AWS are great examples of consumption-based pricing models done right because they give the customer flexibility and focus on minimizing wastage. Unfortunately, not all consumption based pricing is made equal - and there are models designed to maximize profit/ out of convenience.
Consumption-based pricing, we know, that doesn’t work
Although consumption-based pricing is a popular model and varies based on consumption, how it’s delivered isn’t always advantageous for customers.
Take the MAU (Monthly Active Users) model, frequently used by PaaS businesses. Conceptually, the MAU model is simple, based on the total number of unique users in a month. Consumers of this model often like the predictability, since they understand both how many customers (users) they have to service each month, and the cost per customer to provide that service.
But as I will show you, MAU is not a good example of consumption-based pricing optimized for customers. It is in fact optimized for convenience and for maximizing the vendor’s profits, and inherently wastes resources.
MAU pricing demystified
To illustrate how MAU pricing works, and why it’s inherently wasteful at the expense of the customer, I will break down how MAU pricing works for a typical chat use case. The principle applies to all MAU pricing, but I chose chat as it’s top of mind for me with the recent launch of our Ably Chat offering.
Let’s consider a few of the potential underlying costs to operate a chat service with some variability in resources used:
- Maintain a connection to the service for as little as a few minutes, but up to 43k minutes (one month).
- Receive and publish chat messages or reactions, ranging from a few events for private chats to potentially hundreds of thousands per user with popular live stream chat use cases.
- Store messages, attachments, and files for an indefinite amount of time. Not only is there cost to store new data each month, but there are ongoing monthly storage costs for previously stored data that increase monthly, often indefinitely, for each active user.
Using this example, let’s look at why MAU is a flawed consumption-based pricing model:
Problem #1: Consumption waste that the customer pays for
In order for a vendor to price an MAU, they will have to normalize usage of a “typical” user across their entire customer base. They may, for example, allocate up to 100 hours of connection time, 5 thousand messages and 100MiB of storage for each MAU.
Much like the models that came before AWS’s introduction of per second billing by resource, MAU is the modern chat equivalent of renting servers by the month. No single user in a month can ever fit into the “typical” allocation, and will inevitably under-utilize connections hours, messages or storage - yet the customer is paying for the “typical” user.
It’s perhaps easier to visualize this waste by creating a model with a visualization. I have assumed a vendor offers MAU pricing based on a realistic usage model that assumes users are online for 8 hours a day, will send and receive up to one thousand messages per day, and store files up to 100MiB in total. Now let’s assume we have 3 customers with different usage patterns.
- “SaaS biz” has users online for 8 hours a day but only on weekdays. They will consume ~70% of the connection minutes quota. SaaS users consume few messages (50 per day) given the low number of users per “room”, so each user only manages to consume 3.5% of the quota.
- “Live event biz” has 2 events per month for 2 hours, yet these events are busy with 120 messages per minute per user during the event. With users online only 4 hours a month, only 1.6% of the connection quota is used yet 95% of the message quota is used.
- “Customer support biz” end users raise 5 tickets a month, and spend an hour on each publishing and receiving 120 messages. Storage is used for attachments, with each user publishing one 256KiB attachment per ticket. With users online only 5 hours a month, 2% of the connection quota is used, 2% of message quota is used, and this use case uses some of the storage quota but only 7.5% based on an average user being active for 6 months.
As you can see below, no single use case benefits from the MAU model with utilization of the quota very low i.e. wastage is very high.
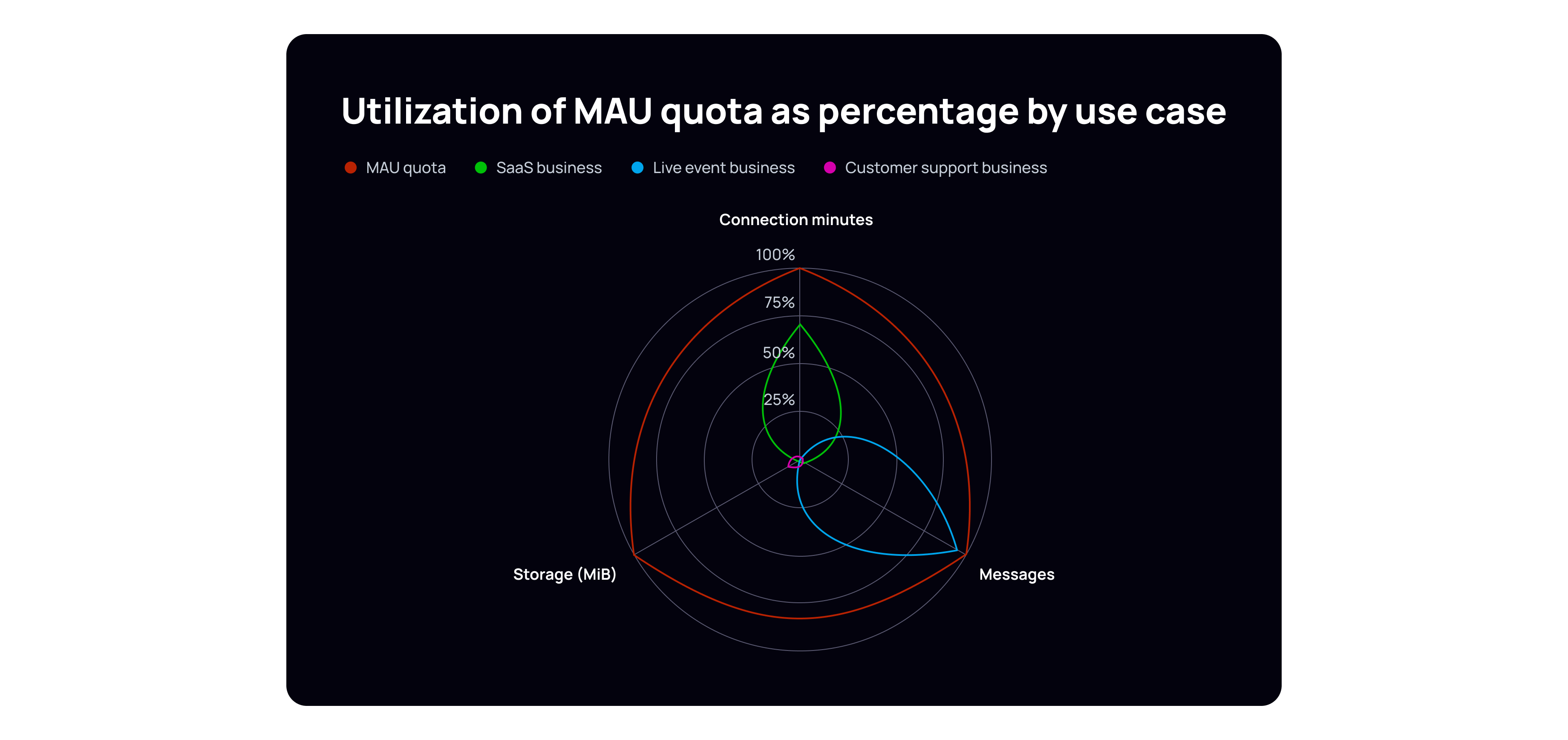
The solution?
Charge customers for their usage based on how users actually consume service resources (resources being connection time, messages, and storage).
Problem #2: Overage charges break the MAU promise of predictable pricing
The sales pitch for MAU is predictable pricing. But this is misleading.
When usage exceeds the “typical” usage for their MAU, most vendors charge for overages. In addition, most vendors have additional consumption units billed in addition to MAU.
Take GetStream for example. They offer unlimited connection time for each MAU. However, they impose a concurrency limit that assumes no more than 5% of all users will be online at any point in time. If you exceed that, each additional concurrent user is billed at 10x the MAU rate. To put that in context, if you ran a popular event where all users joined to watch the finale, customers of GetStream could expect a bill of 95x more than the MAU rate alone.
The solution?
Charge customers for their usage based on how users consume service resources (resources being connection time, messages, and storage).
Problem #3: Costs bloat as no one is incentivized to optimize usage
MAU pricing often encourages the wrong behaviors for customers. The bill at the end of each month is determined by the number of active users alone. Connection time, message and storage usage plays no part in the bill.
Unsurprisingly, customers are not incentivized to optimize usage as a result. In fact, the model can have the opposite effect, encouraging customers to try and “make the most” of their package by using as much as possible.
To illustrate this, when storage is “free” and bundled into every MAU, consumers of the API are likely to store message data indefinitely. This side effect inadvertently creates a vicious cycle of constant price increases based on customer behavior. If storage is “free”, customers will (ab)use it and store data without consideration of the cost. The vendor in turn sees that average storage per MAU is climbing, and will in turn put up their prices to account for more storage. This in turn leads to yet more usage by the customer, and the vicious cycle continues. All of this comes at the expense of the customer.
The solution?
Charge customers for their usage based on how users consume service resources (connection time, messages, and storage).
How we’ve approached consumption-based pricing
Ably’s pricing model is not novel; it’s just designed around what’s best for our customers and builds on the successes of the giants in this space, like AWS and Snowflake. What is surprising, though, is that our pricing model bucks the trend in the chat-as-a-service space, where the MAU model remains predominant.
The principles we apply to our pricing are:
- Customer first: Ably optimizes pricing for the customer’s benefit, not ours. Our success is driven by our customers’ success, and our pricing must align with that.
- Minimize wastage with granular billable unit abstractions: There is no “typical” user for billing purposes. Akin to what AWS did to revolutionize the “typical” server model, we ensure our customers pay for the resources they consume in the most granular way possible. For example, we charge for channel minutes (total number of minutes a channel is connected to Ably in a month), connection minutes (total number of minutes devices are connected to Ably in a month) and messages (total number of messages published and received).
- Economies of scale are shared: Ably is performing trillions of operations for billions of devices each month, which allows us to benefit from economies of scale. Like us, our customers expect to see cost efficiencies as they scale. We operate a transparent model where unit pricing decreases as consumption increases, incentivizing customers to grow with Ably and benefit from cost efficiencies.
- Cost optimization tooling: Customers succeed with Ably when they can increase the value from our service by optimizing spend on billable units. Talking about this principle is not enough, so we invest in features and tooling to help customers with cost optimization. A good recent example of this is the introduction of server-side message batching that can help customers realize cost efficiencies north of 100x compared to MAU.
If all of these pricing principles resonate with you, you can read more about Ably’s new pricing in our announcement blog, in our chat pricing article, or on our pricing page, or just give us a try (we have generous free accounts too).
If, however, you like the predictability of MAU and are not convinced by the problems I’ve outlined above, please reach out to me - I’d love to hear what I’ve missed and consider that as we continue to evolve our pricing.